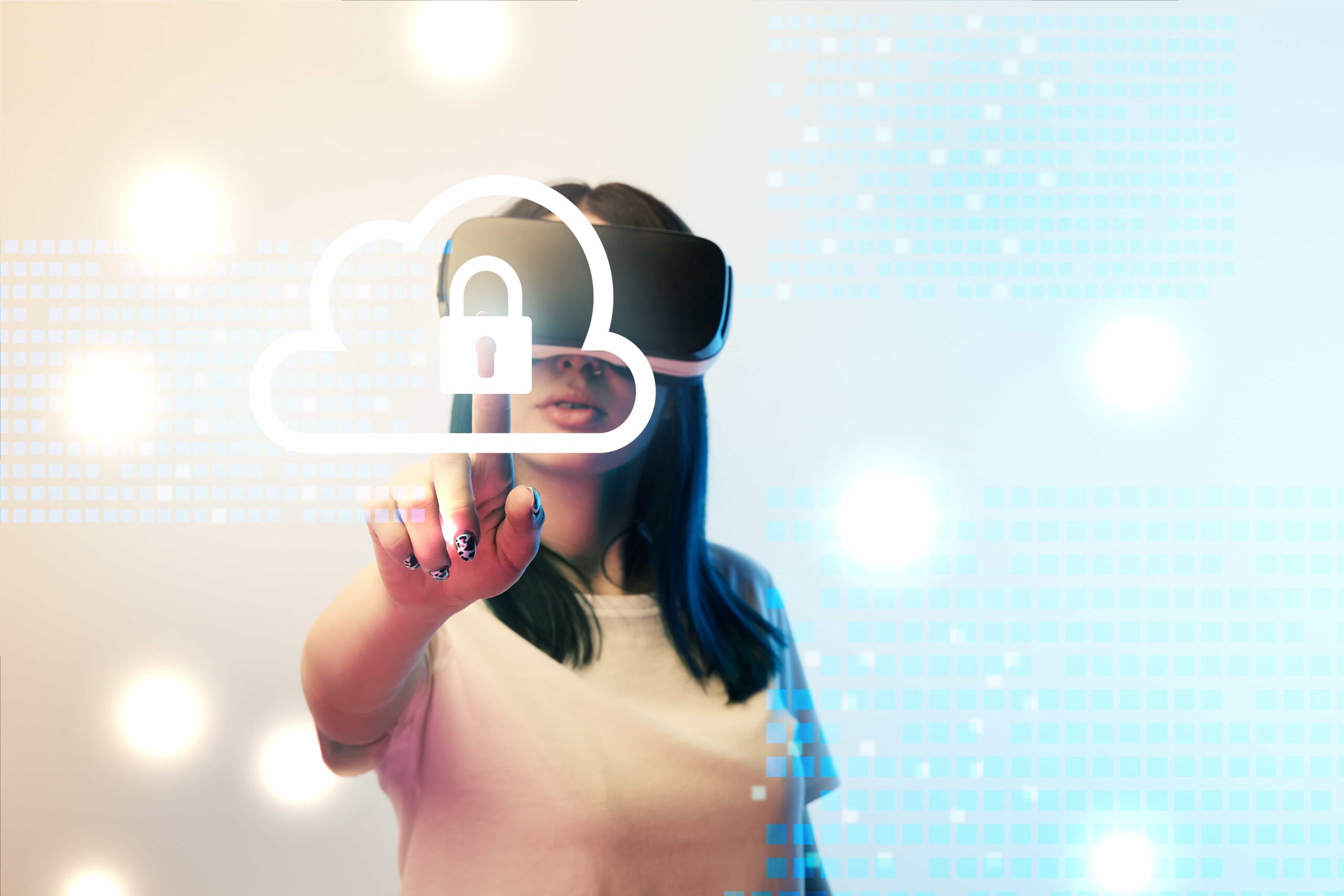
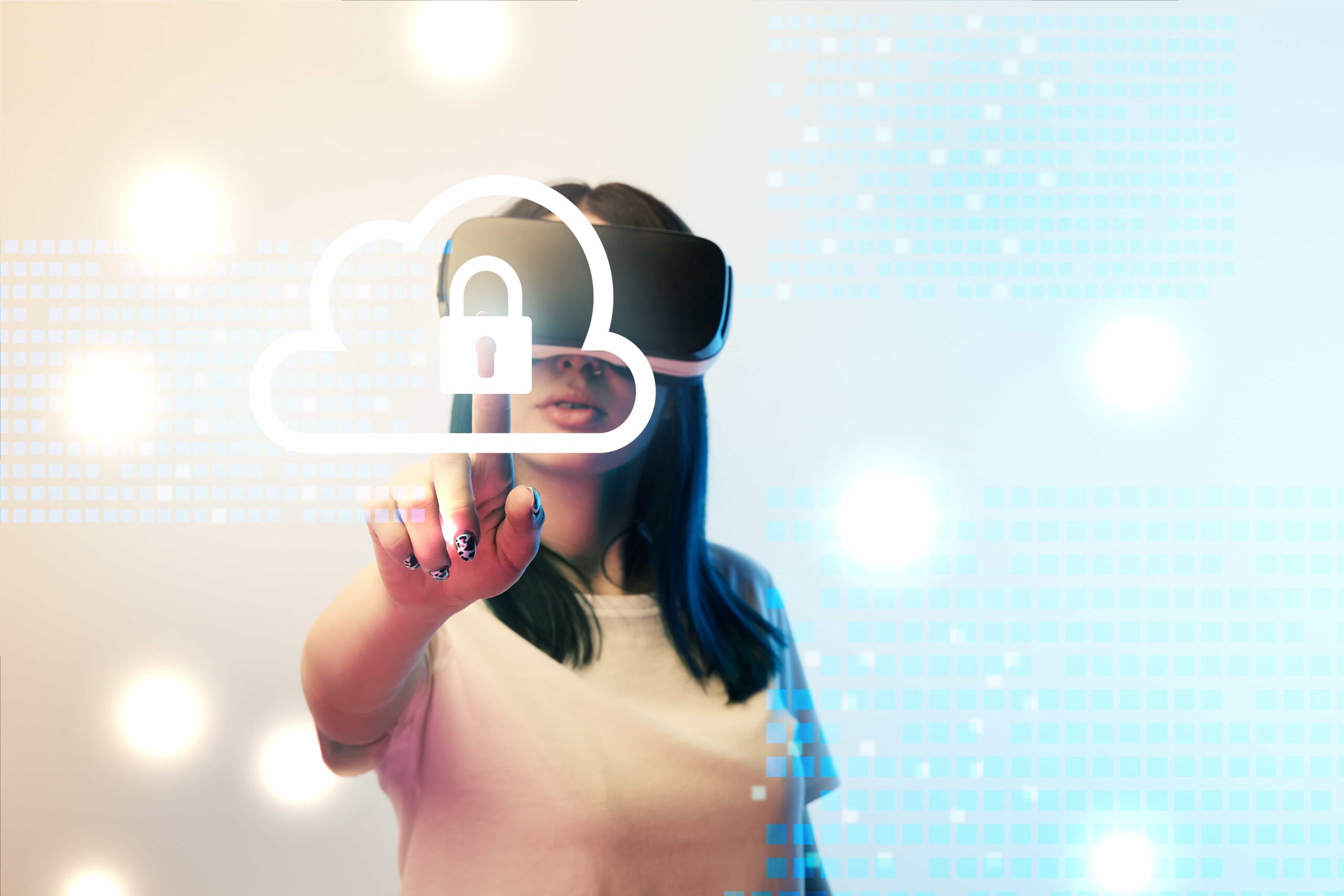
In the rapidly evolving landscape of artificial intelligence, innovative approaches are continually reshaping how we access and process information. Two such groundbreaking technologies are Retrieval Augmented Generation (RAG) and its advanced counterpart, GRAPH RAG. These methodologies integrate AI language models with retrieval systems to deliver highly accurate, context-aware insights. This post explores both ordinary RAG and GRAPH RAG, highlighting how they are transforming the future of information retrieval.
Retrieval Augmented Generation (RAG) is an AI framework that enhances the capabilities of language models by integrating a retrieval component into the generation process. Unlike traditional models that rely solely on pre-trained data, RAG models actively retrieve relevant information from external sources during inference. This fusion allows the model to generate responses that are both contextually rich and grounded in up-to-date information.
Dynamic Knowledge Integration: Accesses external documents or databases in real-time to supplement responses.
Contextual Relevance: Improves the pertinence of answers by focusing on information directly related to the query.
Enhanced Accuracy: Reduces hallucinations and factual errors common in standalone language models.
While ordinary RAG utilizes unstructured text data for retrieval, GRAPH RAG elevates this concept by incorporating knowledge graphs into the retrieval process. Knowledge graphs are structured representations of information where entities and their relationships are mapped out, enabling a deeper understanding of the data.
Structured Data Retrieval: Leverages the interconnected nature of knowledge graphs to retrieve information based on relationships between entities.
Improved Contextual Understanding: Recognizes and utilizes the relationships within data to provide more nuanced and accurate responses.
Efficient Navigation: Traverses complex data landscapes more effectively, leading to faster and more relevant information retrieval.
GRAPH RAG represents a significant leap forward in AI-driven information retrieval, offering a more sophisticated understanding of context and relationships. As knowledge graphs become more prevalent and AI models continue to advance, we can expect:
Interdisciplinary Applications: Combining data from different fields to provide comprehensive insights.
Enhanced Decision-Making Tools: Supporting professionals with complex analysis and predictive capabilities.
Natural Language Understanding: Further closing the gap between human-like understanding and machine processing.
The evolution from ordinary RAG to GRAPH RAG marks a pivotal moment in the development of AI technologies. By integrating retrieval mechanisms with both unstructured and structured data, these systems provide more accurate, context-aware, and insightful responses. Whether you’re a developer, data scientist, or business leader, understanding and leveraging these technologies will be crucial in staying ahead in an increasingly data-driven world.